As global data generation continues to grow exponentially over the last decade, it is predicted to reach 175 zettabytes by 2025. So here's a closer look at understanding the very nature of such vast quantities of "Big-Data" with some relevant tools and techniques to dissect, comprehend and analyze trends and patterns under-the-hood, which could help us take data driven decisions and build predictive models in realms of Science and Technology.
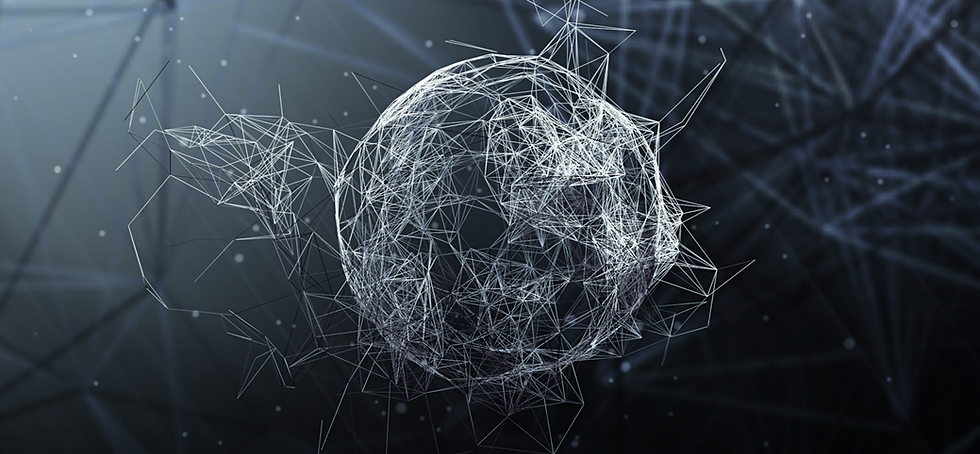
What is Big-Data Analytics?
Big-Data Analytics is the process of examining large and varied data sets that are too large to be stored or analyzed within the framework of a single database or server, in order to uncover hidden patterns, unknown correlations, market trends, customer preferences and other useful information that can help organizations make efficient and informed business decisions.
Big-data is an important data-application concept which is different from the typical structure of a traditional database. With the recent advancements in technologies which support data capturing with high velocity, storage and analysis, the concept of big data has become a widely accepted methodology. Pinning down an exact definition of Big-Data is a bit difficult due to its relatively new and evolving nature. However, the most prevalent one is derived form a famous Gartner report released at the beginning of this millennium.
On this report Big data is defined with 3Vs pertaining to 'volume', 'velocity' and 'variety' and the qualification criteria is met when all 3Vs in a data set are significantly high for traditional analysis techniques . This definition was expanded by Gartner in 2012 introducing 'veracity' into the mix. Data veracity, in general, is how accurate or truthful a data set may be. In the context of big data, however, it takes on a bit more meaning. More specifically, when it comes to the accuracy of big data, it's not just the quality of the data itself but how trustworthy the data source, type, and processing of it is. Also considering that Big data applications should bring incremental 'value' to business, the 5th V, 'value' was added to its definition in 2012 by IDC.
Within the context of this blog we shall deal with the different streams of Big-Data pooled in from the Internet of Things along with its various classifications and respective potential insights in the domains of Science, Technology & Business.
“There were 5 exabytes of information created between the dawn of civilization through 2003, but that much information is now created every 2 days.” - Eric Schmidt (Chairman Alphabet Inc.)
Why is Big-Data Significant in our lives?
Due to the advent of new technologies, devices, and communication means like social networking sites, the amount of data produced by mankind is growing rapidly every year. The amount of data produced by us from the beginning of time till 2003 was 5 billion gigabytes or 5 exabytes as, Eric Schmidt, the ex-chairman of Alphabet puts it. If this entire data dump was piled up in the form of disks it would fill up an entire football field. However, it is surprising to note that same amount of data was created in every two days in 2011, in every ten minutes in 2013 and in every 10 seconds or less in 2018. This rate is still growing enormously enough to reach 175 Zettabytes of data by 2025. Although all this information produced is meaningful and can be useful when processed correctly, it is being neglected without the effective involvement of Artificial Intelligence and Big Data Analytics.
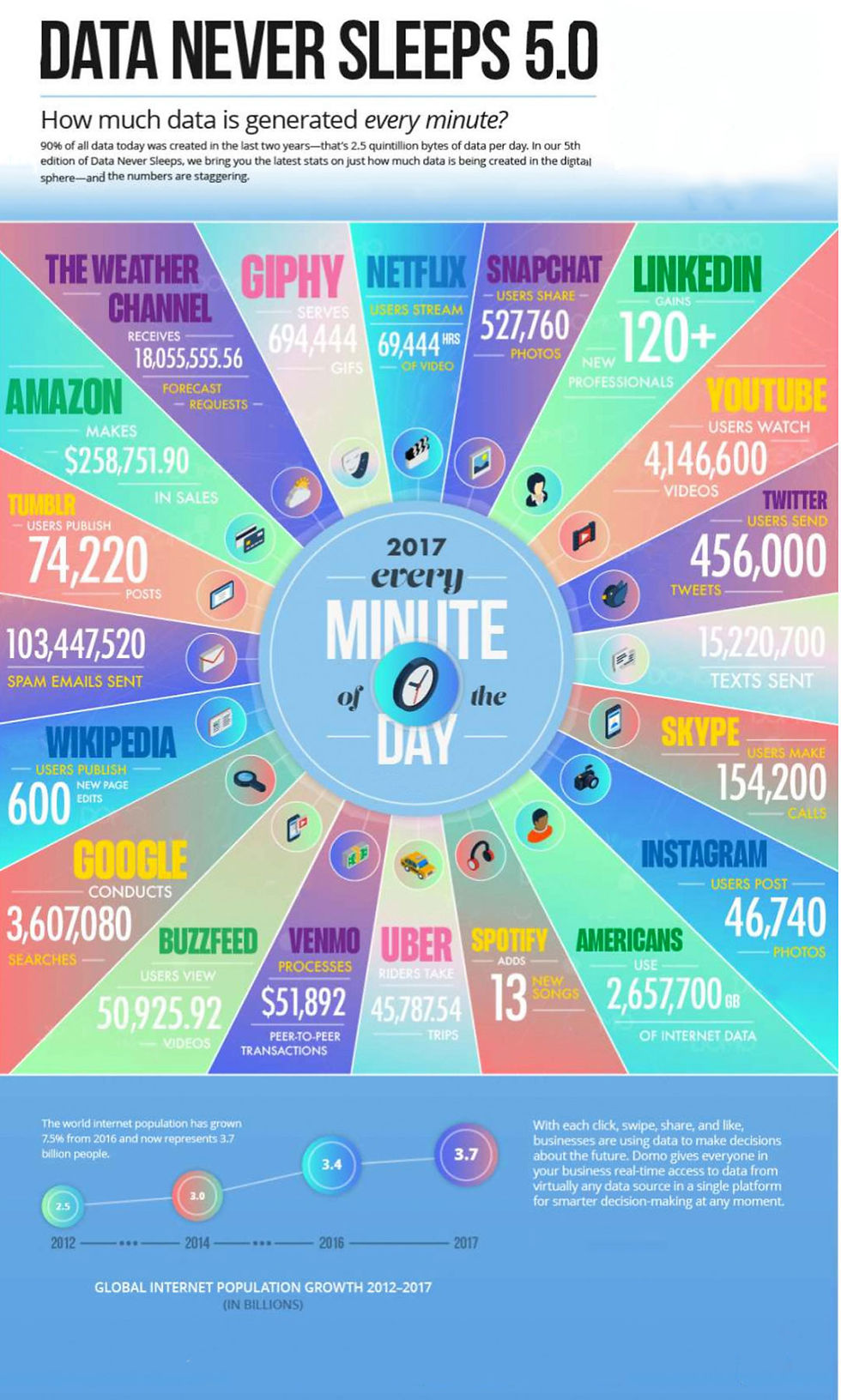
The diagram on the left shows the enormous amount of big-data that was generated on average, every minute of every day throughout different streams and channels of the Internet and the Internet of Things in the year of 2017.
These are mostly Realtime, unstructured streams of data that are in urgent need of classification, categorization and identification for the purpose of finding hidden correlations that might be beneficial for enhancing and simplifying our day to day lives along with boosting business efficiency in quick and effective ways.
This leads us to an enquiry into the various ways that big-data can be useful in bridging this gap using the Hadoop and Kafka ecosystem to make sense of these rapidly expanding streams of data. We shall see that Big-Data can, not only bring about dramatic cost reductions, or new product and service offerings; like traditional analytics, but, it can also support internal business decisions. So far it is evident to us that Big data implies a really large collection of expansive datasets that cannot be processed using traditional computing techniques. Big data is not merely a data, rather it has become a complete subject, which involves various tools, techniques and frameworks. So, it is important for us to understand what can be categorized under this umbrella of Big Data.
The vast Umbrella of Big-Data
Big data involves the data produced by different devices and applications that are broadly categorized under the Internet of Things. Given below are some of the fields that come under the umbrella of Big Data:
Black Box Data: It is a component of helicopter, airplanes, and jets, etc. It captures voices of the flight crew, recordings of microphones and earphones, and the performance information of the aircrafts flying throughout the world.
Social Media Data: Social media such as Facebook and Twitter hold information and the views posted by millions of people across the globe. This includes video feeds, vlogs, images and audio data.
Astronomy & Space Data: Astronomy & Space technologies have been acquiring, systemizing and interpreting large quantities of data through telescopes & satellites for ages. Like many other fields, astronomy has become a very data-rich field, supported by the advances in telescope, computer technology and detector. From large sky surveys, to Dark Energy Surveys & Gravitational Wave Astronomy, the archives and detectors are exploding with Terabytes and Petabytes of data in 2021. Significant volumes of information are also being produced by number of astronomical simulation studies throughout the world . Data mining has ensured the effectiveness and completeness of scientific utilization of such vast and unprecedented datasets leading to a new era of Big-Data Astronomy.
Particle Physics & Bigdata: The quest for understanding the fundamental laws of physics has led scientists far in probing nature at high energies and indeed in exploring the origins of the universe as we observe it. Elusive hints to the Standard Model and new physics are hidden amongst large and complex background processes. The next generations of experiments in particle physics and astro-particles will take an unprecedented large volume of data to achieve their scientific objectives thereby giving rise to Big-Data Particle Physics & Big-Data High Energy Physics in advanced research institutes like CERN.
Stock Exchange Data: The stock exchange data holds information about the ‘buy’ and ‘sell’ decisions made on a share of different companies made by the customers.
Power Grid Data: The power grid data holds information consumed by a particular node with respect to a base station.
Transport Data: Transport data include model, capacity, distance and availability of a vehicle. This also includes live feed and Lidar data from companies like Tesla, Google and Faraday.
Health-Care Data: Health-Care data consists of patient records, health plans, insurance information and other types of information in the form of files and documents, along with data generated from radiological equipment and medical sensors, which can be difficult to manage – but are full of key insights once analytics are applied. It also has the scope of integrating all the real-time data generated by smartwatches, health-bands and the ever-growing streams of data generated by the IoT in the health space.
Sports Data: Sports data includes a large variety of unstructured data with respect to video and image feeds along with structured data in the form of files and documents containing the day-to day stats and medical data of sports personnel and the digital logs of equipment and supplements.
Search Engine Data: Search engines like Google retrieve lots of data from different databases at a tremendous rate, every second of our modern lives.
Furthermore, the very nature of Big-Data can be categorized under 3 broad groups as detailed below:
Structured data: Relational data
Semi Structured data: XML, CSV data
Unstructured data: Word, PDF, Text, Media Logs. (including images, audio and video feeds)
Popular Big-Data Technologies in the Past Decade
Big data technologies developed over the last decade are important in providing accurate analytics from the above sources and classifications, which may ultimately pave the path towards more concrete decision-making, resulting in greater operational efficiencies, cost reductions, and reduced risks in various impactful industrial sections such as Science, Astronomy, Space, Communication, Health, Education etc.
However, in order to harness this power of big data, we would require an infrastructure that can manage and process huge volumes of structured and unstructured data in real-time and can protect data privacy and security.
There are various technologies in the market from different vendors including LinkedIn, Amazon, IBM, Microsoft, etc., to handle Big Data Analytics. However, while looking into these technologies that handle big data, we examine the following two classes of technology:
1) Operational Big-Data
This includes systems like Kafka and MongoDB that provide operational capabilities for real-time, interactive workloads where data is primarily captured and stored. NoSQL Big Data systems are designed to take advantage of new cloud computing architectures that have emerged over the past decade to allow massive computations to be run inexpensively and efficiently. This makes operational big data workloads much easier to manage, cheaper, and faster to implement.
Some NoSQL systems can provide insights into patterns and trends based on real-time data with minimal coding and without the need for data scientists and additional infrastructure.
2) Analytical Big-Data
This includes systems such as the Hadoop Distributed File System, MapReduce and Massively Parallel Processing (MPP) database systems that provide analytical capabilities for retrospective and complex analysis which may span across all of the data.
MapReduce provides a new method of analyzing data that is complementary to the capabilities provided by SQL, and a system based on MapReduce can be scaled up from single servers to thousands of high and low-end machines. These two classes of technology are complementary and frequently deployed together.
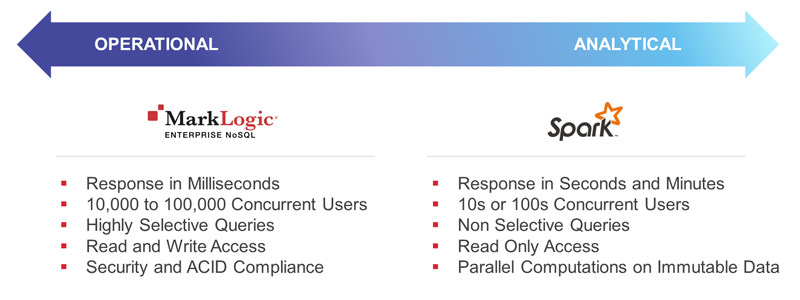
Useful Aspects of Big-Data Analytics
Data management: Data needs to be high quality and well-governed before it can be reliably analyzed. With data constantly flowing in and out of an organization, it's important to establish repeatable processes to build and maintain standards for data quality. Once data is reliable, organizations should establish a master data management program that gets the entire enterprise on the same page.
Data mining: Data mining technology helps us examine large amounts of data to discover patterns in the data – and this information can be used for further analysis to help answer complex business questions. With data mining software, one can sift through all the chaotic and repetitive noise in data, pinpoint what's relevant, use that information to assess likely outcomes, and then accelerate the pace of making informed decisions.
In-Memory Analytics: By analyzing data from system memory (instead of from your hard disk drive), you can derive immediate insights from your data and act on them quickly. This technology is able to remove data prep and analytical processing latencies to test new scenarios and create models; it's not only an easy way for organizations to stay agile and make better business decisions, but, it also enables them to run iterative and interactive analytics scenarios.
So far, we have discussed the variety of categories which fall under the umbrella of Big-Data along with its broad classifications, but it is essential to be clear on what exactly we want to achieve through the big data initiative. What are the business outcomes? What are our expectations? What are the key areas of a business that we would like to enhance using big data analytics? Most of all what are the breakthroughs in software technologies that can provide right answers to these overwhelming problems? Is there a one-stop-solution to all these Big Data Problems?
Stay tuned for the next blog post which introduces artificial intelligence driven, robust and scalable solutions to all such Big Data challenges!
Comments